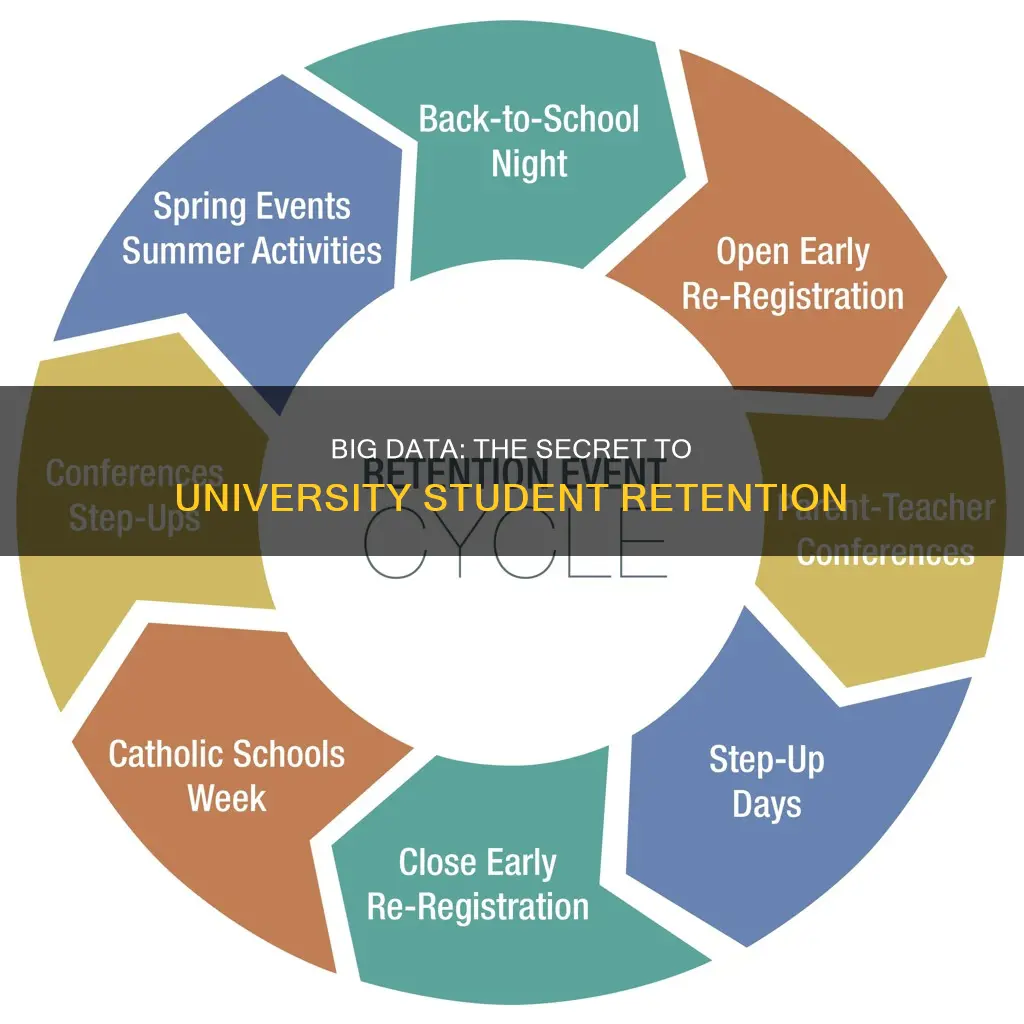
Big data is being used by universities to improve the student experience and keep students enrolled. Universities are using big data to identify talent pools and relevant candidates, as well as to improve the decision-making process and increase efficiency. Big data can also be used to help teachers do their jobs better, as data analytics can be applied to curriculum evaluation and prompt immediate teaching actions. For example, big data can be used to alert administrators when a specific course is experiencing a large number of dropouts, allowing them to investigate the cause of the problem. In addition, big data can be used to guide students toward courses that best align with their strengths, boosting the chances of student success and satisfaction.
Characteristics | Values |
---|---|
Predicting student success | Gannon University applies data analytics to investigate high school performance data, helping the university predict the most suitable courses for incoming students. |
Identifying vulnerable students | UKOU started using data analytics to identify vulnerable students in order to increase retention. |
Improving the quality and effectiveness of educational content | By analyzing data on how students interact with course materials, educators can see what works and what doesn't. This analysis leads to the development of more engaging and effective content. |
Customization of educational content | Big data allows for the customization of educational content to meet diverse learning needs. |
Targeted placement | Using data to gain insights into enrollment processes means educational institutions can guide students toward courses that best align with their strengths. |
Financial analytics | The University of Kentucky uses predictive analytics to improve student retention rates by predicting which students may need financial aid. |
Tracking students' digital footprints | Schools can make calculated decisions about their admissions outreach. |
Predicting student performance | Georgia State University uses a rating system based on how students have performed in marker courses, if they've taken the right courses for their major, and whether they've been flagged for skipping class or flunking quizzes. |
Identifying talent pools | Universities are working hard to identify talent pools to appeal to suitable candidates. |
Curriculum evaluation | Big data can be used to alert administrators when a specific course is experiencing a large number of dropouts, allowing them to investigate the cause of the problem. |
Cost savings | Institutions can use data analytics to cut energy costs and save on operating expenses. |
What You'll Learn
Predicting student success
Big data is being used by universities to predict student success and keep students enrolled. Gannon University, for example, applies data analytics to investigate high school performance data, helping the university predict the most suitable courses for incoming students. This proactive approach to student placement plays a crucial role in setting up students for success from the outset.
Similarly, the University of Kentucky uses predictive analytics to improve student retention rates. They use predictive models to see which students may need financial aid to stay enrolled. Without financial aid, the University of Kentucky's data showed that only 60% of students were likely to continue their studies.
Georgia State University in Atlanta is another example of a university that has successfully used big data to boost student success rates. The university uses predictive analytics to advise students on their choice of major. The university's data analysis showed that many students who didn't get into the nursing major ultimately dropped out of college because they had wasted their first two years taking prerequisites that didn't count toward another major. By steering these students towards a different major early in their college careers, Georgia State has been able to improve graduation rates.
Universities are also using big data to improve the quality and effectiveness of educational content. By analyzing data on how students interact with course materials, educators can see what works and what doesn't. This analysis leads to the development of more engaging and effective content. For example, Arizona State University has taken big data into the classroom to target one of the biggest barriers to student success: mathematics. The university replaced standard lectures on its general-level maths course with a "mathematics emporium".
Big data can also be used to help teachers do their jobs better because feedback happens more quickly and often. With data analytics, teachers can immediately take action to ultimately provide richer learning experiences for students. For example, the University of Leeds has a student lifecycle program that collects data at every stage, from the initial application to student exams and going through their undergraduate, postgraduate, or postdoc training.
University of Detroit Mercy: Student Population and Campus Life
You may want to see also
Identifying vulnerable students
Big data is being used by universities to identify vulnerable students and improve retention rates. Gannon University, for instance, applies data analytics to investigate high school performance data, helping the university predict the most suitable courses for incoming students. This proactive approach to student placement plays a crucial role in setting up students for success from the outset.
The University of Kentucky uses predictive analytics to improve student retention rates. They use predictive models to see which students may need financial aid to stay enrolled. Without financial aid, the University of Kentucky's data showed that only 60% of students were likely to continue their studies.
Georgia State University in Atlanta is another example of a university that uses big data to identify vulnerable students. The university sorts students into three risk categories, colour-coded like a traffic light: green, yellow and red. The system alerted Keenan Robinson, a student who wanted to major in nursing, that he was in the 'yellow' category. The university advised him to abandon nursing as data showed that many students who didn't get into the nursing major ultimately dropped out of college because they had wasted their first two years taking prerequisites that didn't count toward another major.
Big data can also be used to help teachers do their jobs better because feedback happens more quickly and often. Data analytics can be applied to curriculum evaluation, alerting administrators when a specific course is experiencing a large number of dropouts, allowing them to investigate the cause of the problem. With the proper data in hand, the institution can identify whether there is a problem with the standard of teaching, the lecturer, or something else.
Financial Aid for Hampton University Students: How Common?
You may want to see also
Targeted placement
The University of Kentucky uses predictive analytics to improve student retention rates. They use predictive models to identify students who may need financial aid to remain enrolled. Without financial aid, the University of Kentucky's data showed that only 60% of students were likely to continue their studies.
Similarly, Georgia State University in Atlanta uses data analysis to steer students towards courses that are a better fit for them. The university found that many students who didn't get into the nursing major ultimately dropped out because they had wasted their first two years taking prerequisites that didn't count toward another major. By identifying these students early on and guiding them towards a different major, the university can improve retention rates.
Arizona State University is another example of a university that uses big data to improve student placement. The university found that many of its students were coming in unprepared for college-level mathematics, so it replaced standard lectures on its general-level maths course with a "mathematics emporium".
By using data to guide student placement, universities can improve retention rates and set students up for success. This targeted placement strategy is a powerful tool for universities to keep students enrolled and on track to graduation.
Why Non-Adventists Choose Andrews University
You may want to see also
Student lifecycle programmes
Student lifecycle programs are an effective way to collect data at every stage of a student's journey, from the initial application to exams, enrolment, and beyond. The University of Leeds, for example, has been running such a program for several years, collecting data from the application stage, through exams and enrolments, and then from an employability perspective.
The data collected can be used to guide students toward courses that align with their strengths, boosting the chances of student success and satisfaction. Gannon University, for instance, applies data analytics to investigate high school performance data, helping predict the most suitable courses for incoming students. This proactive approach to student placement can set students up for success from the very beginning.
Data can also be used to identify students who may need extra support or additional challenges to reach their full potential. For example, the University of Kentucky uses predictive analytics to improve retention rates by predicting which students may need financial aid to stay enrolled.
Additionally, big data can be used to improve the quality and effectiveness of educational content. By analyzing how students interact with course materials, educators can develop more engaging and effective content that meets diverse learning needs. This tailored approach enhances the overall educational experience and outcomes.
The use of student lifecycle programs and big data analytics allows universities to innovate and ensure they continue to offer highly relevant learning experiences.
Graduating Debt-Free from Columbia University: Is It Possible?
You may want to see also
Curriculum evaluation
Big data is being used by universities to improve the student experience and keep students enrolled. Curriculum evaluation is a key part of this.
Firstly, big data can be used to identify students who are struggling and may need extra support, or those who are not being challenged enough and could benefit from extra assignments. This can be done by collecting data at every stage of the student lifecycle, from application to exams and beyond. This data can be used to create a customised program for each student, allowing them to develop their own personalised program and work at their own pace.
Secondly, big data can be used to improve the quality and effectiveness of educational content. By analysing how students interact with course materials, educators can understand what works and what doesn't, and develop more engaging and effective content. This tailored approach makes content more accessible to a diverse range of learners.
Thirdly, big data can be used to identify courses with high dropout rates, allowing administrators to investigate the cause of the problem. This could be due to the standard of teaching, the lecturer, or another factor. By understanding the reasons behind dropouts, institutions can make changes to the curriculum to better meet the needs of students and keep them enrolled.
Finally, big data can be used to guide students towards courses that best align with their strengths, setting them up for success from the outset. This targeted placement boosts the chances of student success and satisfaction and can help improve retention rates.
Overall, big data plays a crucial role in curriculum evaluation, allowing universities to make data-driven decisions to improve the student experience and keep students enrolled.
University Student Depression: Understanding the Underlying Causes
You may want to see also
Frequently asked questions
Universities are using big data to guide students towards courses that align with their strengths, boosting the chances of student success and satisfaction.
Big data allows universities to identify students who may need financial aid to continue their studies and to customise educational content to meet diverse learning needs.
Big data can be used to predict which students are likely to drop out without financial aid, allowing universities to take action to keep students enrolled.
By analysing data on how students interact with course materials, educators can develop more engaging and effective content that is accessible to a wide range of learners.
Universities are using big data to improve the quality and effectiveness of teaching, to provide a more personalised student experience, and to identify and support talented students who can contribute to the development of the university.